AI-Driven Demand Forecasting in CPG – A comprehensive guide
Accurate demand forecasting is essential in the consumer-packaged goods (CPG) industry. It not only underpins inventory management and production planning, but also reliable financial predictions. It also ensures CPGs have sufficient stock to meet account demand and provides commercial teams with the right data to base their plans on. With the evolution of Artificial Intelligence (AI), a new era of demand forecasting is taking shape, revolutionizing the way CPG companies optimize their operations.
In this comprehensive guide, we will delve into the world of AI-driven demand forecasting for the CPG industry. We’ll explore the technical aspects, challenges, and solutions. Additionally, we’ll examine the user perspective, discover best practices, and analyze real-life examples and common pitfalls in AI-driven forecasting.
Understanding AI in CPG demand forecasting and the technical aspects
AI for CPG demand forecasting is a powerful tool. It leverages advanced algorithms and predictive capabilities to revolutionize traditional approaches to baseline forecasting. Let's break down the details:
Technical complexity
AI algorithms can be intricate, making it challenging for non-technical users to comprehend their inner workings. Moreover, this technical complexity can deter users from trusting AI-driven insights. Addressing this challenge involves providing user-friendly interfaces and clear explanations of the measures in place to ensure the model's reliability and user understanding of the outcomes. Conducting training workshops to empower users with basic knowledge can also be beneficial. Demystifying the "Black Box" is not just about explaining AI concepts but, more importantly, unpacking the metrics and processes that the software employs to make AI insights accessible, alleviating these concerns.
Validation and testing
Users might fear that AI models, being complex, could produce unpredictable results or errors. Which in turn could result in misguided business decisions. To address this concern, implementing rigorous validation and testing processes can enhance the reliability of AI models. Regular audits, validation against historical data, and feedback loops can all help identify and rectify errors promptly, instilling confidence in users.
Maintaining human control
Users might worry about becoming overly reliant on AI, leading to a loss of human control and intuition in decision-making processes. It's crucial to emphasize that AI is a tool to enhance decision-making, not replace it. Encouraging users to interpret AI-driven insights in conjunction with their domain expertise ensures a balanced approach. One where human expertise and intuition and AI-driven predictions complement each other.
Resistance to change
Users might resist adopting AI-based solutions due to a fear of change, especially if existing methods have been in place for a long time. Change management initiatives, user education, and highlighting the benefits of AI-driven forecasting, such as increased accuracy and efficiency, can mitigate resistance. Involving users in the transition process and addressing their concerns can foster a positive attitude toward change.
From a technical perspective, AI-driven demand forecasting relies on sophisticated algorithms and a diverse range of data sources:
Data sources
AI algorithms analyze vast datasets, including customer purchase history, online behavior, social media interactions, market trends, and various external factors. The quality and diversity of these data sources significantly impacts the accuracy of forecasts.
Algorithm complexity
The technical foundation of AI-driven forecasting is rooted in complex machine learning and statistical models. Consequently, the selection of an appropriate algorithm and model complexity play a crucial role in the accuracy of predictions.
Continuous learning
AI models are not static; they can adapt and learn from new data. Therefore, continuous retraining of AI models is essential to account for changing market dynamics and external factors.
User interface
Ensuring user-friendly interfaces is vital for bridging the gap between technical AI capabilities and end-user comprehension. In other words, this point emphasizes usability and the importance of simplicity in gaining insights from AI.
Understanding the technical aspects of AI in demand forecasting in CPG is essential for businesses looking to harness the full potential of these advanced systems. By addressing technical complexity, validation, and user-friendliness, organizations can navigate the intricacies of AI. So that they can leverage it for more accurate and efficient CPG demand forecasting.
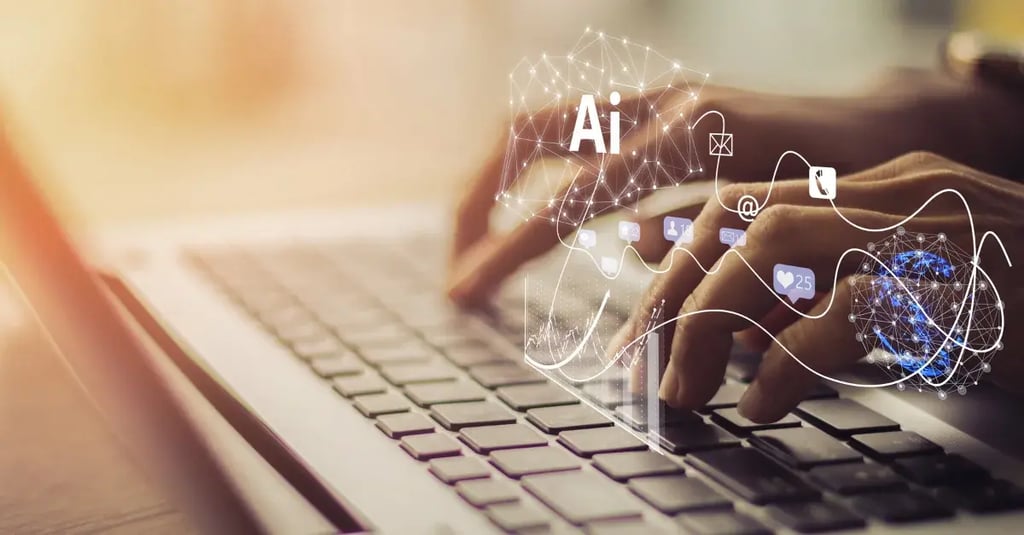
AI forecasting: challenges and solutions from the user perspective
While AI holds immense potential for CPG demand forecasting, adopting it comes with a unique set of challenges, particularly from the user perspective. Users may have concerns, fears, and hesitations that need to be addressed. Let's explore these challenges and discuss practical solutions:
Understanding complex AI algorithms
- Challenge: AI algorithms can be intricate, making it challenging for non-technical users to comprehend their inner workings. As a result, users might fear making decisions based on a system they don't fully understand.
- Solution: Implementing training and workshops can empower users with basic knowledge, making them more comfortable with AI-driven predictions. When complex technical jargon is broken down into understandable terms, users can engage with AI confidently.
Ensuring predictability and reliability
- Challenge: Users might fear that AI models, being complex, could produce unpredictable results or errors, leading to misguided business decisions.
- Solution: Implementing rigorous validation and testing processes can enhance the reliability of AI models. In particular, regular audits, validation against historical data, and feedback loops can help identify and rectify errors promptly, instilling confidence in users. When the accuracy and predictability of AI models is showcased through real-world examples, users can trust AI as a valuable tool in decision-making.
Balancing human control with AI demand forecasting
- Challenge: Users might worry about becoming overly reliant on AI, leading to a loss of human control and intuition in decision-making processes.
- Solution: Emphasizing that AI is a tool to enhance decision-making, not replace it, is crucial. Indeed, encouraging users to interpret AI-driven insights in conjunction with their domain expertise ensures a balanced approach. Because human intuition and AI-driven predictions can complement each other, providing well-informed decisions.
This approach empowers users to leverage AI effectively while maintaining their vital role in the decision-making process.
Overcoming resistance to change
- Challenge: Users might resist adopting AI-based solutions due to a fear of change, especially if existing methods have been in place for a long time.
- Solution: Change management initiatives, user education, and highlighting the benefits of AI-driven forecasting can all mitigate resistance. Involving users in the transition process and addressing their concerns also fosters a positive attitude toward change. Furthermore, demonstrating the increased accuracy and efficiency achieved through AI can help users recognize the advantages and embrace the shift.
Addressing these challenges from the user perspective is crucial for the successful adoption of AI in CPG demand forecasting. By providing support, clear explanations, and real-world results, businesses can help users embrace AI as a valuable tool in enhancing their decision-making processes.
Learn more about Visualfabriq demand forecasting software.
Download the full guide now. Delve into best practices for optimizing revenue predictions, explore real-life examples and use cases, and learn about common pitfalls in AI-driven forecasting in CPG. Download your copy today for actionable insights and guidance.
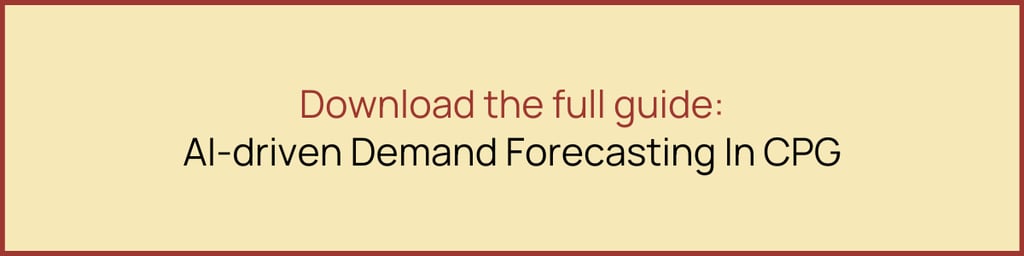